Economic crisis, pandemic, recession…
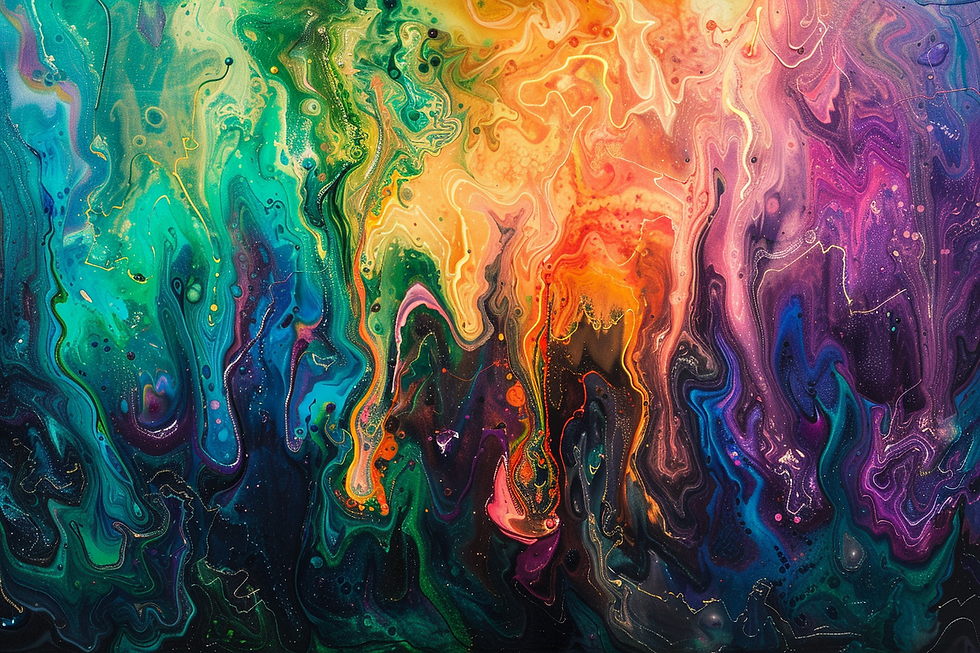
Let’s face it, the last 20 years have been a rollercoaster regarding granting and managing credit. Strategies and predictive models have been affected not only by the usual micro changes that naturally influence portfolios, but also by global events that changed what had been up to that point known and measurable factors. So, the question on everyone’s mind is: will my models still work?
Let’s examine the effects some of these factors have on the performance of models and how organisations can minimise their impact whilst effectively still using the same framework.
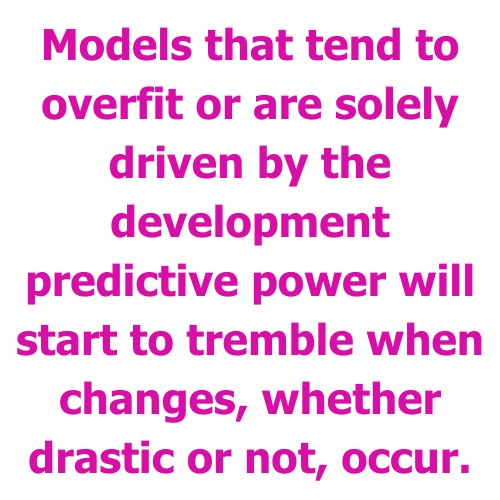
Managing models in a dynamic environment is a challenge that has always been around. Many factors can affect portfolios so, when building models, data scientists must ensure robustness and stability of the models. Models that tend to overfit or are solely driven by the development predictive power will start to tremble when changes, whether drastic or not, occur.
This blog has been split into two parts. This blog deals with effects driven by internal factors. Part Two discusses how external factors may affect the portfolio, strategies, and models and how an organisation may counteract their effects and remain profitable.
Before I go into the various factors, just a word regarding model performance. Many people who use models think that if things change, the model will stop working completely.
This is not the case.
Models may lose predictive power in certain situations, but patterns remain the same. For example, people who didn’t pay in the past will still pose a higher risk than people who did. There will be more non-payers and so distributions change. Models may lose some predictive power, but to completely stop working, these patterns would have to reverse. Understanding how much power may be lost is key to ensuring models continue to be used optimally and avoid unnecessarily returning to a manual process. To gain this understanding, tracking is, as always, extremely important.
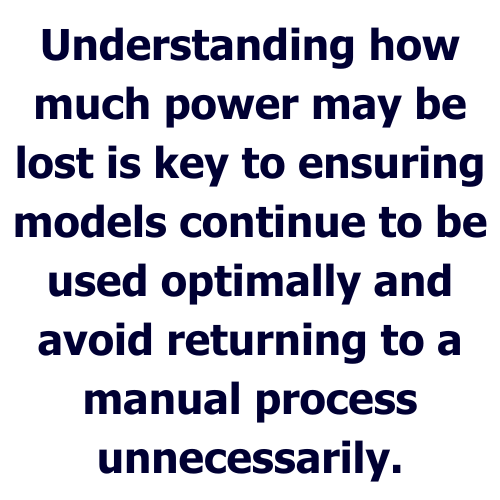
So, let’s review the internal factors data scientists need to ensure they cater for:
Marketing strategy and population shifts
The marketing strategy may change, bringing different profiles and populations to the new accounts area. This will influence the ability of the scorecard to differentiate risk but may also only change the odds observed at each level.
Business objectives may be too ambitious, and this may drive the marketing department to open some of those segments that prove riskier.
If the odds of the population are lower, you may want to increase the resources in your collections department and increase your provision levels to adjust to the new quality of the portfolio. Careful analysis of the vintages AND the score-to-odds relationship will enable you to react swiftly.
If the model was not developed on these type of accounts, it certainly may require development. In most cases, though, a change in cut-off and strategy may suffice.
Product changes: terms and conditions
This may be changes to term duration, interest, or credit limit policies, whether more or less aggressive.
I recently redeveloped a model for a client in Asia where the previous scorecard was not rank ordering at all. This is quite rare, so I reviewed the previous model documentation. The model was full of product features not decided by the customer; but by the bank itself.
The client was changing the product terms and focusing on existing customers and additional loans to them, so the model never had a chance.
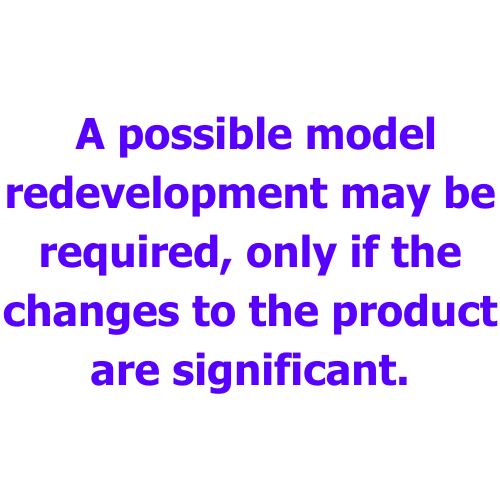
Effective data scientists should not allow this to occur. The model must avoid being affected by the organisation’s practices in such a drastic way. Don’t take me wrong; some of these features are fine, but we can’t let them overpower our models.
If the model is sufficiently robust, then the organisation needs to understand the objectives for the changes. A possible redevelopment may be required only if the changes to the product are significant.
Existing Accounts
Although the existing accounts are not directly affected by the front-end model unless it’s due to new accounts' effects, what occurs in the back end influences the resulting odds of the population.
Modifying the credit limit strategies will influence the overall profitability and also increase the likelihood of customer delinquency. This is due to the additional pressure put on customers’ affordability when spend increases.
Marketing actions may drive spend or balance up through incentives such as vouchers. Or you may decide to change payment terms or interest, which may drive affordability up or down.
You should be able to observe the changes in roll rate reports. Making sure you track changes separately and doing proper A/B testing to understand the effect of strategies before fully implementing the changes is very important to keep control.
If the odds are lowered by these strategies, perhaps consider cut-off changes in the front-end to lower the exposure of the riskier accounts.
Systems
There are many changes that may occur in the systems which will affect the ability the scorecard has to rank order risk. This can range from simple application form changes to full-blown implementations of new systems or process reviews. It may also be the enhancement of the tools used. For example, a new dialler to improve collections.
Whatever the change, most tracking reports will help you identify the effect if you are able to pinpoint what period is affected. A good chronology log will provide the necessary information to determine the course of action needed, whether cut-off changes or redevelopment.
Whilst models must be redeveloped as often as required, they should be able to support internal changes if their builders made them sufficiently robust. Understanding how the changes affect their performance can easily be done by tracking, resulting in possible changes to strategy in a timely manner.
As always, if you have any queries on model building and performance, or you just want to discuss your specific needs, please email us or book a 30 min online meeting with us.
Until next time…
Comments